Achieving Higher Patient Data Integrity Requires a Multi-Layered Approach
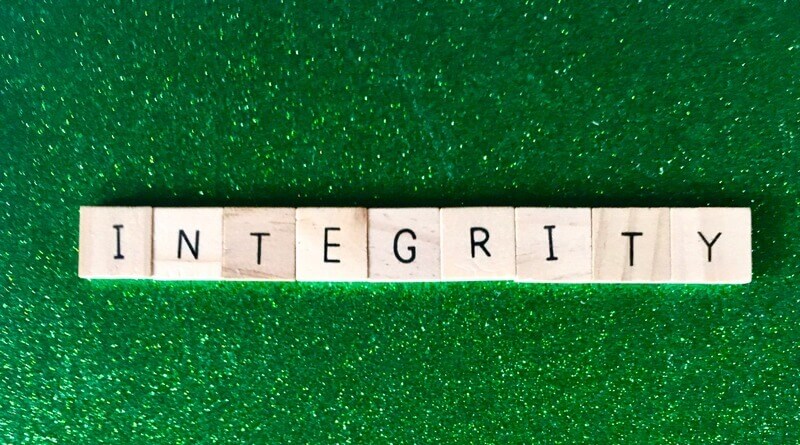
The following guest post was written by David Cuberos, Enterprise Sales Consultant with RightPatient®
Patient Data Integrity and Duplicate Medical Records
It is a well known fact that inaccurate or incomplete data within a patient’s medical record can be a catastrophic risk to patient safety, not to mention a serious hospital liability. As a result, many hospitals and healthcare organizations across the industry are closely examining the integrity of their health data and taking steps to clean it, most by using third party probabalistic and deterministic de-duplication matching algorithms (often directly from their EHR providers) that search and identify possible duplicates for an automatic or manual merge.
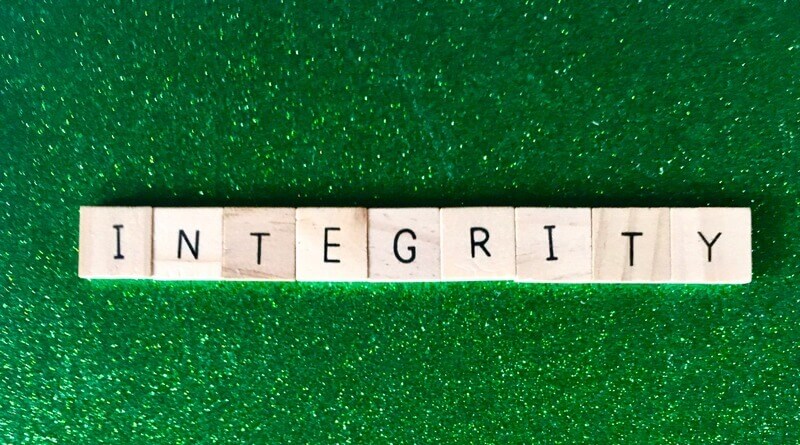
Improving patient data integrity in healthcare requires a multi-layered approach that addresses both data matching and more accurate patient identification.
Several key players in the healthcare industry including CHIME, AHIMA, HIMSS, and major EHR providers are beating the drum to improve patient identification and patient data matching, all important catalysts for the push to improve patient data integrity.
If you are a hospital or healthcare organization that is knee deep in the middle of a health IT initiative to help increase patient data integrity (especially in the context of prepping for participation in a local or regional health information exchange), you may want to stop and reassess your strategy. The rush to cleanse “dirty data” from EHR and EMPI databases is often addressed by relying on an EHR vendor’s de-duplication algorithm which is supposed to search and identify these duplicate medical records and either automatically merge them if similarity thresholds are high, or pass them along to the HIM department for further follow up if they are low.
This could be a very effective strategy to cleanse an EMPI to ensure patient data accuracy moving forward, but is it enough? Is relying on an EHR vendor’s de-duplication algorithm sufficient to achieve high levels of patient data integrity to confidently administer care? It actually isn’t. A more effective strategy combines elements of a strong de-duplication algorithm with strong patient identification technology to ensure that patient data maintains its integrity.
Duplicate Medical Record Rates are Often Understated
The industry push for system-wide interoperability to advance the quality and effectiveness of healthcare for both individuals and the general population has been one of the main catalysts motivating healthcare organizations to clean and resolve duplicates but it also has revealed some kinks in the data integrity armor of many different medical record databases. Most hospitals we speak with either underestimate their actual duplicate medical rate, or do not understand how to properly calculate it based on the actual data they can access. An AHIMA report entitled “Ensuring Data Integrity in Health Information Exchange” stated that:
“…on average an 8% duplicate rate existed in the master patient index (MPI) databases studied. The average duplicate record rate increased to 9.4% in the MPI databases with more than 1 million records. Additionally, the report identified that the duplicate record rates of the EMPI databases studied were as high as 39.1%.”
“High duplicate record rates within EMPI databases are commonly the result of loading unresolved duplicate records from contributing MPI files. EMPI systems that leverage advanced matching algorithms are designed to automatically link records from multiple systems if there is only one existing viable matching record. If the EMPI system identifies two or more viable matching records when loading a patient record, as is the case when an EMPI contains unresolved duplicate record sets, it must create a new patient record and flag it as an unresolved duplicate record set to be manually reviewed and resolved. Therefore, if care is not taken to resolve the existing EMPI duplicate records, the duplicate rate in an EMPI can grow significantly as additional MPI files are added.”
(AHIMA report, “Ensuring Data Integrity in Health Information Exchange” http://library.ahima.org/xpedio/groups/public/documents/ahima/bok1_049675.pdf)
Clearly, the importance of cleansing duplicate medical records from a database cannot be understated in the broader scope of improving patient data integrity but relying on an EHR vendor’s probabilistic matching algorithm as the only tool to clean and subsequently maintain accurate records may not always be the most effective strategy. Instead, healthcare organizations should consider a multi-layered approach to improving patient data integrity beyond relying exclusively on an EHR vendor’s de-duplication algorithm. Here’s why.
Why Patient Data Integrity is a Multi-Layered Approach
Often not clearly explained to healthcare organizations, EHR de-duplication algorithms allow end users to set matching thresholds to be more or less strict, which comes with trade-offs. The more strict the threshold is set, the less chance of a false match but the higher chance of a false reject. The less strict the algorithm is set, the lower the chance of a false reject but the higher the chance of false acceptance.
Translation: Often times hospitals who say they have a low duplicate medical record rate might have a strict false acceptance rate (FAR) threshold setting in their de-duplication algorithm. That may mean that there could be a significant amount of unknown duplicate medical records that are being falsely rejected. Obviously, this is a concern because these databases must be able to identify virtually every single duplicate medical record that may exist in order to achieve the highest level of patient data integrity.
So, what can healthcare organizations do to ensure they are not only holistically addressing duplicate medical record rates, but also adopting technology that will maintain high patient data integrity levels moving forward? One answer is to implement a stronger de-duplication algorithm that has the ability to “key” and link medical records across multiple healthcare providers on the back end, and deploying a technology such as biometrics for patient identification on the front end to ensure that not only is care attribution documented to the accurate medical record, but a provider has the ability to view all patient medical data prior to treatment.
For example, many credit bureaus offer big data analytics solutions that can dig deep into a medical record database to better determine what identities are associated with medical records. These agencies are experts in identity management with access to sophisticated and comprehensive databases containing the identification profiles for millions and millions of patients — databases that are reliable, highly accurate, and secure with current and historical demographic data.
Once data is analyzed by these agencies, they are able to assign a “key” to match multiple medical records for the same patient within a single healthcare organization and across unaffiliated healthcare organizations to create a comprehensive EHR for any patient. Offering a unique ability to augment master patient index (MPI) matching capabilities with 3rd party data facilitates more accurate matching of medical records across disparate health systems and circumvents the problem of MPIs assigning their own unique identifiers to individual patients that are different than unaffiliated healthcare organizations that have their own MPI identifiers.
Benefits of using a third party big data analytics solution that has the ability to “key” medical records for more accurate patient data matching at a micro level include:
- More accurate identification of unique patient records resulting in a more complete medical record and improved outcomes
- Prevention of duplicate medical records and overlays at registration reduces the cost of ongoing MPI cleanups
- Medical malpractice risk mitigation
- Reduced patient registration times
- The ability to more accurately link the most current insurance coverage patient information for more accurate billing
On the marco level, benefits include:
- Positive patient identification for eligibility verification, billing, coordination of benefits, and reimbursement
- Improved care coordination
- Information and record keeping organization
- Linkage of lifelong health records across disparate healthcare facilities
- Aggregation of health data for analysis and research
- Accurately aggregating patient federated data via a HIE
Conclusion
We have long championed the idea that improving patient data integrity can never be achieved in the absence of establishing patient identification accuracy or relying on EHR vendor de-duplication algorithms as the single resource to clean an MPI database. Hospitals and healthcare organizations that are truly committed to cleansing duplicate medical records from their databases and preventing them from reoccurring through more accurate patient identification must consider deploying stronger front and back end solutions that have the ability to more comprehensively identify and resolve these dangers to patient safety. Why not leverage the clout and reach of these big data analytics solutions to more effectively improve patient data integrity instead of putting all of your eggs in an EHR vendor’s de-duplication algorithm?
What other strategies have you seen as effective methods to increase patient data integrity in healthcare?
David Cuberos is an Enterprise Sales Consultant with RightPatient® helping hospitals and healthcare organizations realize the benefits of implementing biometrics for patient identification to; increase patient safety, eliminate duplicate medical records and overlays, and prevent medical identity theft and healthcare fraud.
Great post, thanks for sharing. Data-driven decision making will be one of the key factors in changing the future of healthcare. There is so much great work being done with data analysis and data linkage tools in other industries as well, It will be interesting to see the impact of these changes down the road.
Simon Emmitt
http://DataLadder.com