The Difference Between 1:N, 1:1, and 1:Few and Why it Matters in Patient ID
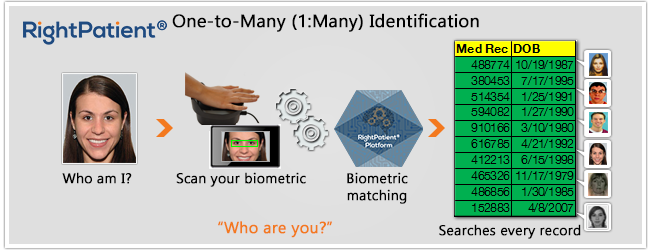
The following guest post was submitted by Joe Kubilius, Director of Product and Process Management at RightPatient®
Understanding the Digital World
In a world rife with digital devices and electronic gadgets, few of us probably know or understand how they actually work. Think about a smartphone for example – myriad buttons, switches, cameras, lights, and sounds instruct us to swipe this, or press that and we oblige. After all, the complexity of the backend processor and sensor network that makes these devices do what they do is probably of little interest to most of us, myself included.
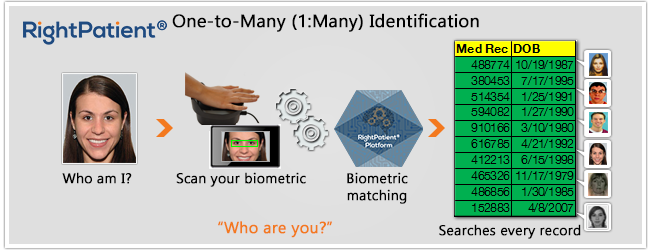
Did you know that not all biometric patient ID systems have backend matching types that can prevent duplicates, eliminate medical ID theft, and improve patient data integrity? Only 1:N biometric matching has this capability.
Instead what we focus on is the end result – what you see, hear, and experience when you use a digital device. Few would argue that it’s necessary or even mandatory to have a thorough understanding of backend functionality on any digital device in order to appreciate the value it brings to our lives. For biometric patient identification solutions, this is definitely not the case.
Why Biometric Patient ID Technology is Different
Understanding biometric matching types is critical when selecting a patient identification solution. Most of us probably see biometric matching as rather black and white — for example, you place your finger on a fingerprint reader, a backend software program recognizes and verifies your identity, and you are on your merry way. The problem is that backend biometric matching technology is not cookie cutter and there are different matching types that carry different capabilities.
Why is this important to know and understand? We know that most healthcare organizations invest in the use of biometric patient ID solutions to increase patient safety by:
- Eliminating medical identity theft and fraud at the point of service
- Preventing duplicate medical records and overlays
- Achieving and sustaining patient data integrity
- Safeguarding personal health information (PHI)
- Identifying unconscious or unknown patients
What most people don’t realize is that depending on which biometric matching type you select, achieving these goals is not 100% attainable with select patient ID solutions. The ONLY way to achieve the bulleted objectives is to implement a system that, during patient enrollment, compares a patient’s stored biometric template against ALL stored templates in the biometric database. If the ultimate goal is to improve patient safety and patient data integrity, only a one-to-many (1:N) biometric matching type can accomplish this.
Let’s take a closer look at the available biometric matching types and what they have the ability to do.
Understanding the Differences Between Biometric Matching Types
Biometric matching types can be categorized as: One-to-many or “Identification” (1:N), one-to-one or “Verification” (1:1), and 1:Few Segmented “Identification” (1:Few). Here is a breakdown of each matching type and how to interpret their capabilities:
- (1:1) Verification: 1:1 biometric “verification” matching authenticates a patient’s identity by comparing a captured biometric template with a biometric template pre-stored in a database. 1:1 biometric matching rejects or accepts a patient’s identity but before the comparison takes place, hospital staff must first input a personally identifiable credential (e.g. – a date of birth, gender, etc.) prior to comparing a stored biometric template against a live scan. This personally identifiable credential points to a specific enrollment template in the database so using a 1:1 matching type answers the question, “Is a patient who they claim to be.”
Example: A patient walks into the ED. Hospital staff asks the patient for their date of birth then scans the patient’s biometric credential to compare it against the stored template for that patient to verify that the patient is who they claim to be. With 1:1 biometric matching, the registrar has to retrieve a patient’s medical record first. Assuming the patient has been previously enrolled, they then scan their biometric and the system compares the captured data only against the data on file for that medical record.
Takeaway: 1:1 biometric verification is beneficial for verifying a patient’s claimed identity but since it does not search the stored biometric template database in its entirety, it does not have the ability to prevent medical identity theft or fraud at the point of service nor does it have the capability to identify an unconscious or unknown patient since a personally identifiable credential is needed prior to conducting the biometric scan.
- (1:Few) Segmented Identification: 1:Few biometric matching compares a patient’s captured biometric template against a segmented portion of the entire biometric database, therefore a personally identifiable credential must be provided prior to the biometric scan to determine the subset of biometric templates to compare against. For example, a patient would provide a date of birth prior to the biometric scan and a 1:Few segmented identification system would then compare that patient’s biometric template only against the templates that share the same date of birth.
Example: A patient arrives at a medical facility for treatment. At registration, hospital staff asks the patient for their date of birth which segments the biometric database to only those records that share the same date of birth and then captures the patient’s biometric credential for comparison against the segmented database.
Takeaway: 1:Few segmented identification does not have the ability to search an entire biometric database in real time to prevent the creation of duplicate medical records or eliminate medical identity theft or fraud at the point of service. What if a patient attempting to commit fraud had previously enrolled their biometric credentials and it was linked to another electronic medical record, then returns to the medical facility claiming another identity and providing a falsified, different date of birth? Hospital staff would then link that patient’s biometric credentials to another electronic medical record and a 1:Few segmented identification matching type would not be able to catch the fraud or prevent a duplicate medical record for this patient. In addition, if a patient arrived unconscious without any identification credentials in the ED, 1:Few segmented identification does not have the ability to identify them because a personally identifiable credential is required. How would an unconscious, unknown patient be able to provide this? Biometric patient ID matching systems based on 1:Few segmented identification do not have the ability to identify unconscious/unknown patients.
- (1:N) Patient Identification: A one-to-many (1:N) biometric identification matching system instantly compares a patient’s captured biometric template against ALL stored biometric templates in the system. No other information is required from the patient other than their biometric credentials and this matching type represents the only true de-duplication mechanism and the only way to prevent duplicate medical records to achieve and sustain patient data integrity. 1:N biometric mathcing types ensure that once a patient enrolls, it is impossible to create a duplicate medical record for that patient.
Example: A patient arrives at a hospital for outpatient surgery. At the registration desk, hospital staff takes a patient’s photo with an iris recognition camera. The backend software instantly compares that patient’s biometric credentials to every single stored biometric template in the database.
Takeaway: 1:N biometric matching is the only true way to prevent duplicate medical records and overlays and eliminate medical identity theft and healthcare fraud at the point of service. By searching the ENTIRE biometric enrollment template database, hospital staff ensures that a patient has not tried to claim another patient’s identity, and is able to access the only electronic medical record linked to that patient with confidence. If a healthcare organization seeks to improve and sustain patient data integrity and patient safety, 1:N biometric searches are the only way to accomplish this. In our 1:Few example above, if an unconscious, unknown patient arrived in the ED and a hospital had implemented a biometric patient ID system with 1:N matching, hospital staff would only need to capture the patient’s biometric credential for accurate identification.
Understanding the capabilities and limitations of biometric matching types is key to select a biometric patient ID system that will accomplish the goals of improving patient safety and patient data integrity in healthcare. Take the time to ask the right questions when evaluating a biometric patient ID solutions so you won’t be left in the dark about what a solution can and can’t achieve.
Have you implemented a biometric patient ID system based on 1:1 or 1:Few segmented matching type and did not understand the limitations? Please share your comments and feedback below!
Joe Kubilius is Director of Product and Process Management with RightPatient®. With over 10 years of experience in the design, development, and implementation of biometric identity management solutions, Joe has been integral to the success of hundreds of large and small scale deployments across the globe.
Nice observation. Good Information Joe Kubilius..